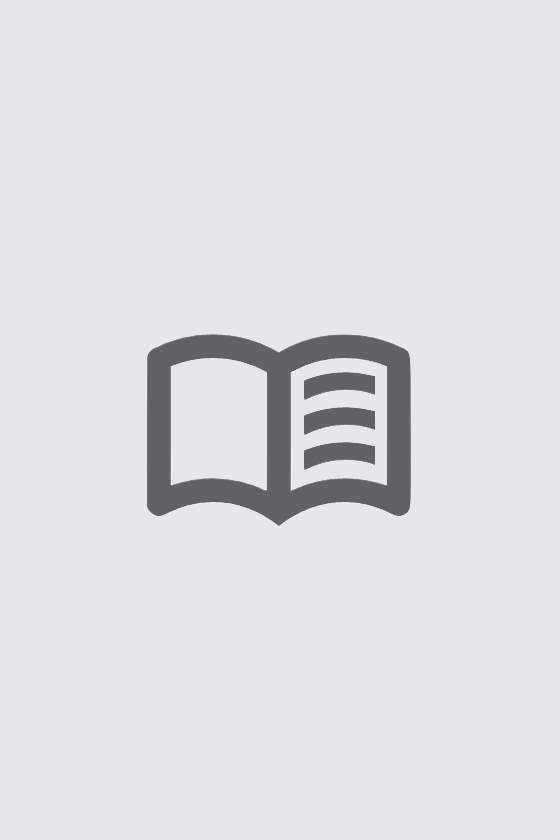
From Complex Sentences to a Formal Semantic Representation using Syntactic Text Simplification and Open Information Extraction e-bog
436,85 DKK
(inkl. moms 546,06 DKK)
This work presents a discourse-aware Text Simplification approach that splits and rephrases complex English sentences within the semantic context in which they occur. Based on a linguistically grounded transformation stage, complex sentences are transformed into shorter utterances with a simple canonical structure that can be easily analyzed by downstream applications. To avoid breaking down th...
E-bog
436,85 DKK
Forlag
Springer Vieweg
Udgivet
29 august 2022
Genrer
CFX
Sprog
English
Format
pdf
Beskyttelse
LCP
ISBN
9783658386979
This work presents a discourse-aware Text Simplification approach that splits and rephrases complex English sentences within the semantic context in which they occur. Based on a linguistically grounded transformation stage, complex sentences are transformed into shorter utterances with a simple canonical structure that can be easily analyzed by downstream applications. To avoid breaking down the input into a disjointed sequence of statements that is difficult to interpret, the author incorporates the semantic context between the split propositions in the form of hierarchical structures and semantic relationships, thus generating a novel representation of complex assertions that puts a semantic layer on top of the simplified sentences. In a second step, she leverages the semantic hierarchy of minimal propositions to improve the performance of Open IE frameworks. She shows that such systems benefit in two dimensions. First, the canonical structure of the simplified sentences facilitates the extraction of relational tuples, leading to an improved precision and recall of the extracted relations. Second, the semantic hierarchy can be leveraged to enrich the output of existing Open IE approaches with additional meta-information, resulting in a novel lightweight semantic representation for complex text data in the form of normalized and context-preserving relational tuples.