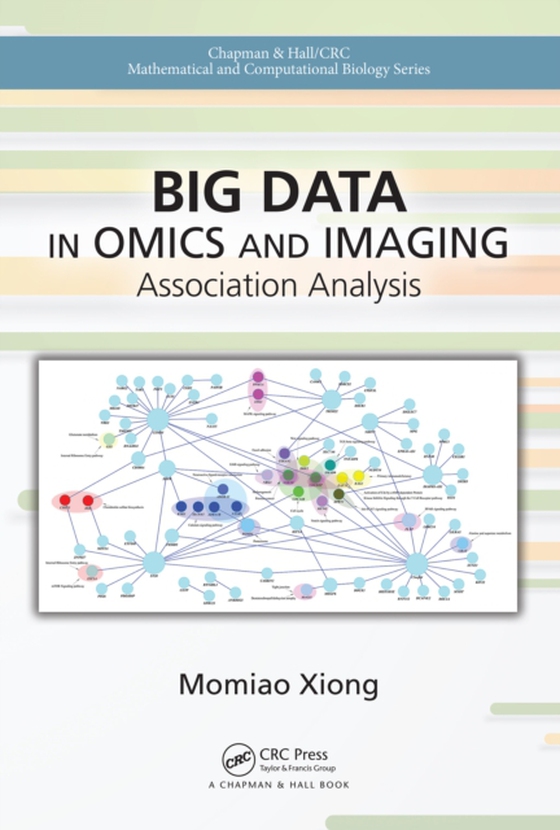
Big Data in Omics and Imaging e-bog
403,64 DKK
(inkl. moms 504,55 DKK)
Big Data in Omics and Imaging: Association Analysis addresses the recent development of association analysis and machine learning for both population and family genomic data in sequencing era. It is unique in that it presents both hypothesis testing and a data mining approach to holistically dissecting the genetic structure of complex traits and to designing efficient strategies for precision m...
E-bog
403,64 DKK
Forlag
Chapman and Hall/CRC
Udgivet
1 december 2017
Længde
668 sider
Genrer
Probability and statistics
Sprog
English
Format
epub
Beskyttelse
LCP
ISBN
9781315353418
Big Data in Omics and Imaging: Association Analysis addresses the recent development of association analysis and machine learning for both population and family genomic data in sequencing era. It is unique in that it presents both hypothesis testing and a data mining approach to holistically dissecting the genetic structure of complex traits and to designing efficient strategies for precision medicine. The general frameworks for association analysis and machine learning, developed in the text, can be applied to genomic, epigenomic and imaging data.FEATURESBridges the gap between the traditional statistical methods and computational tools for small genetic and epigenetic data analysis and the modern advanced statistical methods for big dataProvides tools for high dimensional data reductionDiscusses searching algorithms for model and variable selection including randomization algorithms, Proximal methods and matrix subset selectionProvides real-world examples and case studiesWill have an accompanying website with R codeThe book is designed for graduate students and researchers in genomics, bioinformatics, and data science. It represents the paradigm shift of genetic studies of complex diseases- from shallow to deep genomic analysis, from low-dimensional to high dimensional, multivariate to functional data analysis with next-generation sequencing (NGS) data, and from homogeneous populations to heterogeneous population and pedigree data analysis. Topics covered are: advanced matrix theory, convex optimization algorithms, generalized low rank models, functional data analysis techniques, deep learning principle and machine learning methods for modern association, interaction, pathway and network analysis of rare and common variants, biomarker identification, disease risk and drug response prediction.a