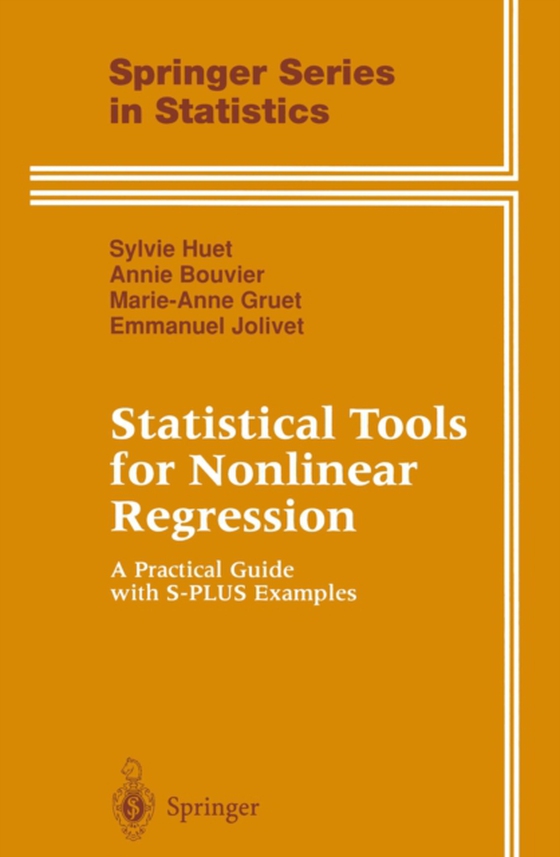
Statistical Tools for Nonlinear Regression e-bog
692,63 DKK
(inkl. moms 865,79 DKK)
If you need to analyze a data set using a parametric nonlinear regression model, if you are not on familiar terms with statistics and software, and if you make do with S-PLUS, this book is for you. In each chapter we start by presenting practical examples. We then describe the problems posed by these examples in terms of statistical problems, and we demonstrate how to solve these problems. Fina...
E-bog
692,63 DKK
Forlag
Springer
Udgivet
17 april 2013
Genrer
Probability and statistics
Sprog
English
Format
pdf
Beskyttelse
LCP
ISBN
9781475725230
If you need to analyze a data set using a parametric nonlinear regression model, if you are not on familiar terms with statistics and software, and if you make do with S-PLUS, this book is for you. In each chapter we start by presenting practical examples. We then describe the problems posed by these examples in terms of statistical problems, and we demonstrate how to solve these problems. Finally, we apply the proposed methods to the example data sets. You will not find any mathematical proofs here. Rather, we try when possible to explain the solutions using intuitive arguments. This is really a cook book. Most of the methods proposed in the book are derived from classical nonlinear regression theory, but we have also made attempts to provide you with more modern methods that have proved to perform well in practice. Although the theoretical grounds are not developed here, we give, when appropriate, some technical background using a sans serif type style. You can skip these passages if you are not interested in this information. The first chapter introduces several examples, from experiments in agron- omy and biochemistry, to which we will return throughout the book. Each example illustrates a different problem, and we show how to methodically handle these problems by using parametric nonlinear regression models.