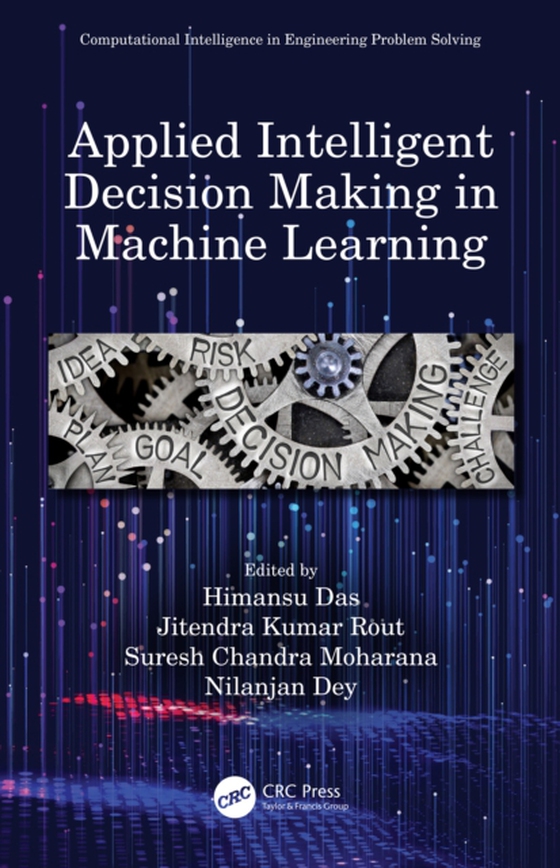
Applied Intelligent Decision Making in Machine Learning e-bog
546,47 DKK
(inkl. moms 683,09 DKK)
The objective of this edited book is to share the outcomes from various research domains to develop efficient, adaptive, and intelligent models to handle the challenges related to decision making. It incorporates the advances in machine intelligent techniques such as data streaming, classification, clustering, pattern matching, feature selection, and deep learning in the decision-making process...
E-bog
546,47 DKK
Forlag
CRC Press
Udgivet
18 november 2020
Længde
252 sider
Genrer
THR
Sprog
English
Format
pdf
Beskyttelse
LCP
ISBN
9781000208542
The objective of this edited book is to share the outcomes from various research domains to develop efficient, adaptive, and intelligent models to handle the challenges related to decision making. It incorporates the advances in machine intelligent techniques such as data streaming, classification, clustering, pattern matching, feature selection, and deep learning in the decision-making process for several diversified applications such as agriculture, character recognition, landslide susceptibility, recommendation systems, forecasting air quality, healthcare, exchange rate prediction, and image dehazing. It also provides a premier interdisciplinary platform for scientists, researchers, practitioners, and educators to share their thoughts in the context of recent innovations, trends, developments, practical challenges, and advancements in the field of data mining, machine learning, soft computing, and decision science. It also focuses on the usefulness of applied intelligent techniques in the decision-making process in several aspects. To address these objectives, this edited book includes a dozen chapters contributed by authors from around the globe. The authors attempt to solve these complex problems using several intelligent machine-learning techniques. This allows researchers to understand the mechanism needed to harness the decision-making process using machine-learning techniques for their own respective endeavors.